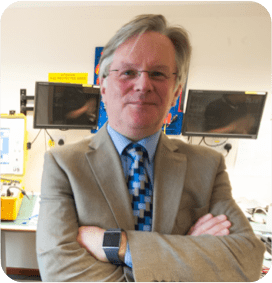
Before investing in an IoT system, take a step back and ask yourself some basic questions, says Jim Cockram, CENSIS Business Development Manager
It’s difficult to not get excited about the Internet of Things (IoT). After all, it is the main driving force behind the fourth industrial revolution.
However, the revolution we are faced with is very distinct from its predecessors in many ways. Perhaps foremost among them, it is not a single entity that we can pinpoint and say: “that is when it began”. As action-provoking as talk of revolution might be, it will actually be an extended period of time characterised by the application of existing technologies in incremental steps towards a visionary end goal, rather than one specific giant leap.
For engrained industry looking to the future, it’s important to embrace a stepped approach. The gradual nature of the transition towards the next phase of industry is something manufacturers should keep in mind. As they look to implement new IoT technologies and systems in their businesses, they should look at it as a process of evolution over revolution. In fact, in many cases, it always seems a return to basics is a good starting point.
- Get away from the silver-bullet mentality
The first, and certainly the most important, step is having the right mindset; followed closely by a shared vision. Rather than thinking ‘the answer is technology’, it is more about ‘what is the question that needs answering?’ and certainly not the silver-bullet approach.
While the panacea of engineering is pure prognostics, it is important to strive toward this end-state rather than jump straight to it. Understanding the data you have, how to capture the data you don’t have, and then mastering diagnostics, is the foundation of prognostics. Of course, now the unscheduled downtime is being tackled, there is a need to also understand constraints and to ask: “is there a piece of equipment, or process causing serious operational issues?” Ultimately, it comes down to determining what is the root cause that might be compromising your business in terms of cost, quality, and delivery. There may be a simple answer and the solution might not require a massive capital investment or a sophisticated piece of technology – it could be as simple as upskilling staff or changing a process.
For example, one production line among four other similar production lines I encountered had an issue with productivity, with some staff struggling to keep pace with their colleague. Upon inspection, it transpired there were four people labelling the products in different ways. The business thought it needed a technological solution to solve the problem, but it was merely a case of ensuring that there was a standardised approach, specially adapted point-of-use tools and a bit of upskilling. The key was understanding what the problem actually was, engaging with the operators to exploit their expertise, and then taking the right steps to address it.
- Don’t collect data for data’s sake
Data, we’re now often told, is a business’s most important asset, and in reality, it can be. But why use the word ‘can’? Data is only a small part of the story. A lot of businesses collect a lot of data, in some cases terabytes of data per day, and some don’t collect any data or what they do collect is scant. Collecting data for data’s sake is expensive and it can become a burden. It’s all about what question(s) need answering and how we make the data decision-ready. Collecting the right data by exploring where the information-gaps are, how they can be filled, and how the data can be normalised, standardised and collated to make it fit for analytics; this is all harder than it sounds, but it is fundamental in making data work for you.
Once data is answering the foremost questions posed, it then exposes more information gaps, and so the iterative cycle begins. It is important to fix problems and get things moving, but there is a second-class activity that falls by the way-side, and that is root cause analysis; data capture and analytics to help focus the team on fixing the problem right first time and then, ultimately, eliminating the problem altogether. Now the data is an asset!
- It’s not about the ‘obvious data’
Some may say that overheating of a device needs to monitored for temperature, and have an alert set at a designated threshold. However, other parameters may factor in the device overheating, and these might not be obvious. It may need some thought and correlation as to the contributing factors. One specific problem that I was asked to look at was an intermittent tripping of electrical circuit breakers. The data that was recommended to be collected ranged from time of day, to current load and voltage spikes – all of which is fairly obvious. However, of less evident relevance was the weather: rain was a significant factor because the factory’s earthing point was a local ground earth which can be affected by moisture in the soil.
Generally speaking, data collection can be a highly mandrolic process – it requires a lot of manual work and human intervention. However, once you know what data you need and where to capture it, this can be automated, especially with the accessibility, cost effective and easy exploitable IoT devices or systems now available.
- It’s an iterative process
Like any change management activity, it’s very much an iterative process. Implementing IoT systems, capturing the data, analysing it, acting on it, and reflecting on its benefits isn’t a one-time event: you have to decide what the priorities by doing a criticality analysis (as my mentor says to me all the time: “what is eating your lunch!”), and so tackle the project step by step. In that respect, the process is never truly complete, but one of continuous improvement.
Introducing change might increase productivity and may reduce machine downtime, and so if the related process becomes more efficient this may have a knock-on effect. A bottleneck might well appear somewhere else and so overnight the ‘old’ call for more staff, more spares and better machinery may well remain anecdotal and be a distraction to what is now the new challenge.
Therefore, make sure the continuous improvement journey is carefully navigated and that data is collected to paint the picture of the before and after. This picture will not only help to reflect the return on investment, but also be able to counter the history chit-chat that may well be a distraction. It is important to take a holistic view rather than looking at isolated problems – adding ‘more’ to a particular operation may only cause more problems and create more bottlenecks.
Before jumping into investing in an IoT system, take a step back and reflect on what questions need to be answered, for what reason and for what effect. Most parameters and imagery, combined with some fairly inexperience processing and cloud services, is all ‘doable’, but the output needs to be translated into Decision Ready Outputs.